After all, people regularly use www.google.com to check if their Internet connection is set up correctly.
— JC van Winkel, Site Reliability Engineering pg. 25
Site Reliability Engineering is a collection of essays written by senior engineers at Google describing how they run their production systems. It's mostly within the context of the Site Reliability Engineering (SRE) organization, which is an actual job title there. However, I found the subject matter to be quite wide-ranging, everything from people management to distributed consensus algorithms. It didn't focus strictly on the SRE discipline, which partly explains why it's 500 pages long.
The whole book is actually available for free online if you're interested in reading it. Or just parts of it, since each chapter is a separate topic and there's not much overlap between them.
In essence, the SRE organization is a specialized discipline within Google meant to promote and maintain system-wide reliability for their services and infrastructure.
Reliability is such a multi-faceted objective that the expertise and responsibilities required are wide-ranging. The end goal seems simple to explain: Ensure systems are operating as intended. But reaching that goal requires a combination of technical, operational, and organizational objectives. As a result, this book touches on basically every topic of interest for a software company.
I spent a couple years working in a Nuclear Power Plant, so I've seen what peak reliability and safety culture looks like. The consequences of errors there are so much higher compared to most other companies, including Google. So it's not a surprise that reliability and safety are the paramount objectives and they take priority over everything else.
This safety culture permeated everything we did within the plant, and around it. There were lines painted on each row of the parking lot to indicate the path to the entrance. If you didn't follow them, you would get coached and written up by someone. It was intense. And don't even think about not holding the railing while taking the stairs either...
Any changes you want to make to a system within the plant needs extensive documentation, review, and planning before being approved. Thus, the turnaround on any change takes months, if not longer.
Contrast that with software companies like Google where 1000s of changes are made on a daily basis. The consequences of a mistake can still be serious, depending on the application. But instead of aiming for no errors, errors are managed like a budget, and the rate at which this budget is spent determines how much change can be made in a given period of time:
In effect, the product development team becomes self-policing. They know the budget and can manage their own risk. (Of course, this outcome relies on an SRE team having the authority to actually stop launches if the SLO is broken).
— Marc Alvidrez, pg. 51
Learning about Google's software development process was interesting. In the first few chapters, there was a lot of useful information on measuring risk, monitoring, alerting, and eliminating toil. These were some of the more insightful chapters in my opinion.
But there were also a few...less insightful chapters. Chapter 17 was about testing code and it was really just stating obvious things about writing tests; it wasn't specific to SRE at all. Then there was a lot of time spent on organizational stuff, like postmortem culture and how to have effective meetings. So much of the writing came off as anecdotal and rather useless advice that the author tried to generalize (or just make up) from past experiences.
So there were good and bad parts of the book. I wouldn't recommend reading it cover to cover like I did. It'd be better to just read a chapter on a topic that's relevant for you.
For instance, I found the section on load balancing to be really informative. Below is a summary of how Google does load balancing.
Balancing Act
Chapters 19 and 20 are about how Google handles their global traffic ingress. Google, by operating one of the largest distributed software systems in the world, definitely knows a thing or two about traffic load balancing. Or to put it in their words:
Google’s production environment is—by some measures—one of the most complex machines humanity has ever built.
— Dave Helstroom, pg. 216
Melodrama aside, I appreciated the clear and concise breakdown of their networking and traffic management in these chapters.
Load balancing needs to consider multiple measures of quality. Latency, throughput, and reliability are all important and are prioritized differently based on the type of request.
1. DNS
Chapter 19 is about load balancing across datacenters. Google runs globally replicated systems, so figuring out which datacenter to send a particular request to is the first step in traffic management. The main mechanism for configuring this is via DNS — a.k.a the phone book of the internet.
The goals of this routing layer are twofold: - Balance traffic across servers and deployment regions fairly - Provide optimal latency for users
DNS responses can include multiple IP addresses for a single domain name, which is standard practice. This provides a rudimentary way of distributing traffic, as well as increasing service availability for clients. Most clients (i.e. browsers) will automatically retry requests to different records in the DNS response until they successfully connect to something. The downside is that the service provider, Google, has little control over which IP address actually gets chosen in the DNS response. So it can't be solely relied on to distribute traffic.
The second goal of DNS is to provide optimal latency to users, which means trying to route their requests to the geographically closest server available to them. This is accomplished by having different DNS name-servers set up in each region Google operates in, and then using anycast routing to ensure the client connects to the closest one. The DNS server can then serve a response tailored to that region.
This sounds great in theory, but in practice DNS resolution is more hairy and there's lots of issues specifically around the caching introduced by intermediary name-servers. I won't go into those details here.
Despite all of these problems, DNS is still the simplest and most effective way to balance load before the user’s connection even starts. On the other hand, it should be clear that load balancing with DNS on its own is not sufficient.
— Piotr Lewandowski, pg 240
2. Reverse Proxy
The second layer of load balancing happens at the "front door" to the datacenter—using a Network Load Balancer (NLB), also known as a reverse proxy. These handle all incoming requests by broadcasting a Virtual IP (VIP) address. Then it can proxy incoming requests to any number of actual application servers. In order retain the originating client details after proxying a request, Google uses Generic Routing Encapsulation (GRE) which wraps the entire IP packet in another IP packet.
There's some complexity here, of course, in terms of the actual routing algorithm used by the NLB. Supporting stateful protocols like WebSockets requires the NLB to keep track of connections and forward all requests to the same backend for a given client session.
Once the request has reached an application server, there will likely be a multitude of internal requests initiated in order to serve the request.
In order to produce the response payloads, these applications often use these same algorithms in turn, to communicate with the infrastructure or complementary services they depend on. Sometimes the stack of dependencies can get relatively deep, where a single incoming HTTP request can trigger a long transitive chain of dependent requests to several systems, potentially with high fan-out at various points.
— Alejandro Forero Cuervo, pg. 243
And besides that, there's plenty of requests and computational work that aren't originated by end-users. Cronjobs, batch processes, queue workers, internal tooling, machine learning pipelines, and more are all different forms of load that must be balanced within a network. That's what Chapter 20 covers.
3. Connection Pool Subsets
The goal of internal load balancing is mostly the same as for external requests. Latency is still important, but the main focus is on optimizing compute and distributing work as efficiently as possible. Since there's only so much actual CPU capacity available, it's vital to ensure load is distributed as evenly as possible to prevent bottlenecks or the system falling over due to a single overloaded service.
Within Google, SRE has established a distinction between "backend tasks" and "client tasks" in their system architecture:
We call these processes backend tasks (or just backends). Other tasks, known as client tasks, hold connections to the backend tasks. For each incoming query, a client task must decide which backend task should handle the query.
— Cuervo, pg. 243
Each backend task or service can be composed of 100s or 1000s of processes in a single machine. Ideally, all backend tasks operate at the same capacity and the total wasted CPU is minimized.
The client tasks will hold persistent connections to the backend tasks in a local connection pool. Due to the scale of these services, it would be inefficient for every single client to hold a connection to every single backend task, because connections cost memory and CPU to maintain.
So Google's job is to optimize an overlapping subset problem—which subset of backend tasks should each client connect to in order to evenly spread out work.
Using random subsetting didn't work. The graph below shows the worst backend is only 63% utilized and the most is 121% utilized.
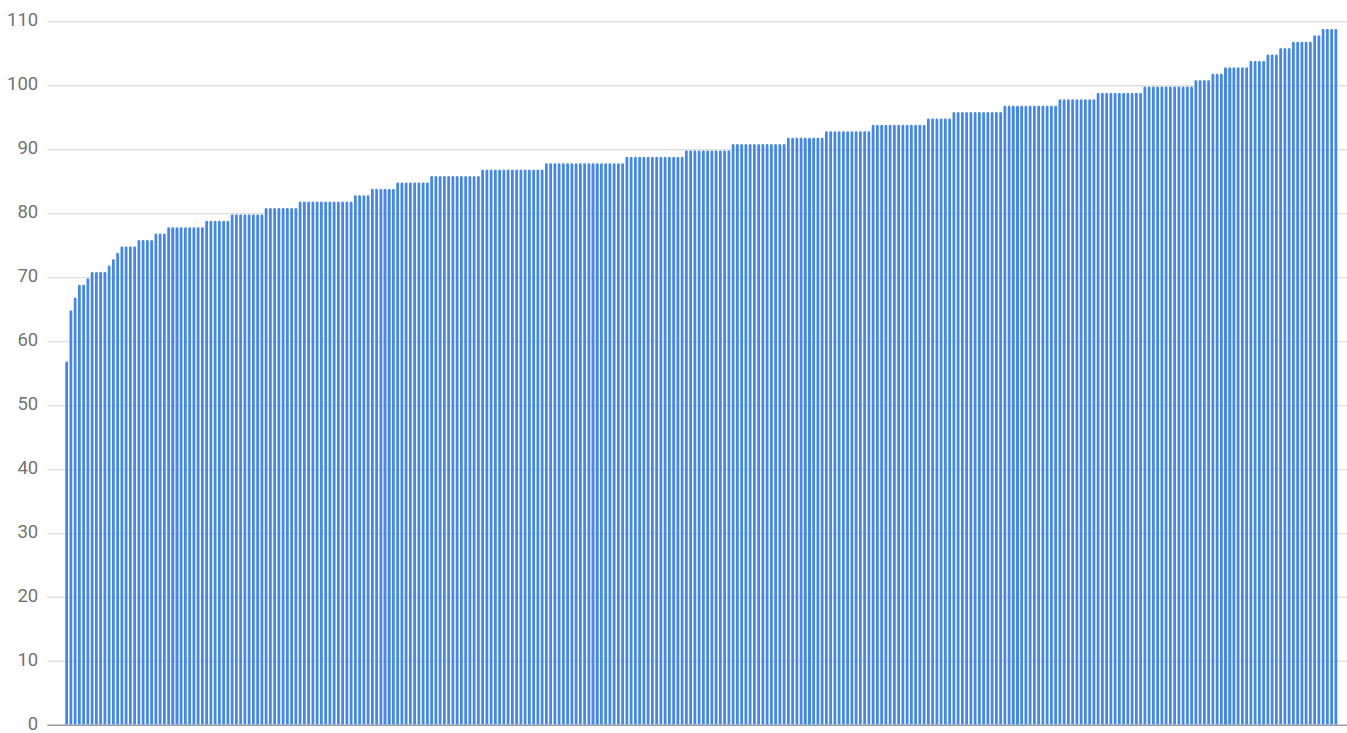
Instead, Google uses deterministic subsetting which perfectly balances the connections between clients. It's an algorithm that shuffles and assigns backends to each subset evenly. Again, I won't go into detail about it.
4. Weighted Routing
Once the pool of connections has been established for each client task, the final step is to build an effective load balancing policy for these backends.
Using a simple round robin algorithm didn't work, as evidenced from historical operational data. The main reason is because different clients will issue requests to the same backends at vastly different rates, since they could be serving completely different downstream applications. There may also be variation in the cost of different queries, backend machine diversity, and unpredictable factors like antagonistic neighbours.
Instead, Google uses weighted round robin which keeps track of each backend's current load and distributes work based on that. First they built it based on active requests to each backend, but this also doesn't tell the whole story of how healthy a particular backend is. So instead, each backend sends load information to the client in every response. It includes active request count, CPU, and memory utilization. The client uses this data to distribute the flow of work optimally.
Here's a diagram I made to visualize all that:
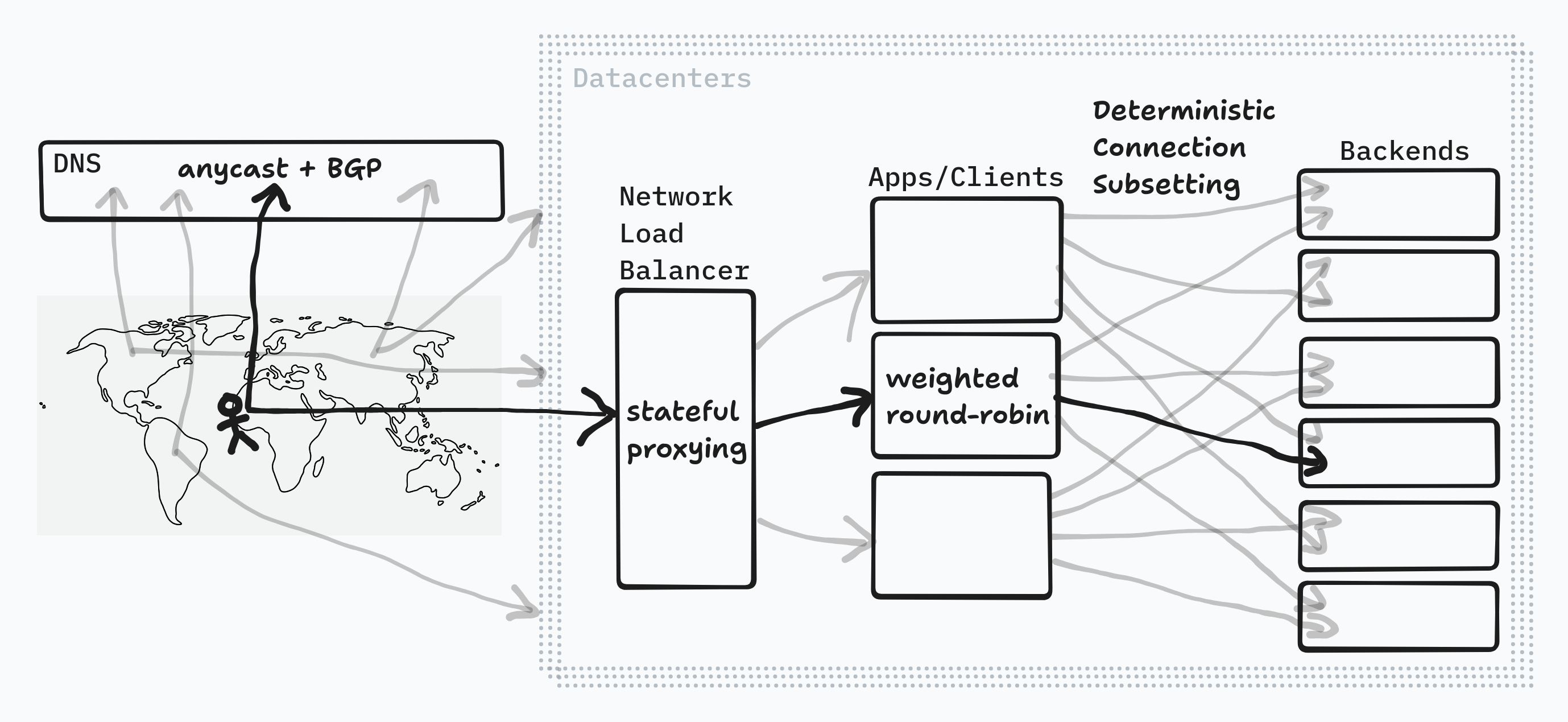
Conclusions
Site Reliability Engineering offers many insights shared by senior engineers from one of the world's leading software companies. I particularly enjoyed the sections on alerting, load balancing, and distributed computing. But there were some chapters I found boring and without much useful, actionable advice.
Google has been a leader and innovator in tech for many years. They're known for building internal tools for basically every part of the production software stack and development life cycle. A lot of these tools have been re-released as open source libraries, or even new companies started by ex-Googlers.
For instance, Google has been running containerized applications for over 20 years. As the scale of running services and jobs this way expanded, the manual orchestration and automation scripts used to administer these applications became unwieldy. Thus, around 2004 Google built Borg — a cluster operating system which abstracted these jobs away from physical machines and allowed for remote cluster management via an API. And then 10 years later, Google announced Kubernetes, the open source successor to Borg. Today, Kubernetes is the de-facto standard for container orchestration in the software industry.
All this to say—Google has encountered many unique problems over the years due to its sheer complexity and unprecedented scale; it's forced the company to develop novel solutions. As such, it's helpful looking to them as a benchmark for the entire software industry. Understanding how they maintain their software systems is helpful for anyone looking to improve their own.